Detecting Human Interpretable Features to Support Poverty Mapping
ENHANCING THE EFFECTIVENESS OF SOCIAL SAFETY NETS
THROUGH AI and MACHINE-LEARNING INNOVATION
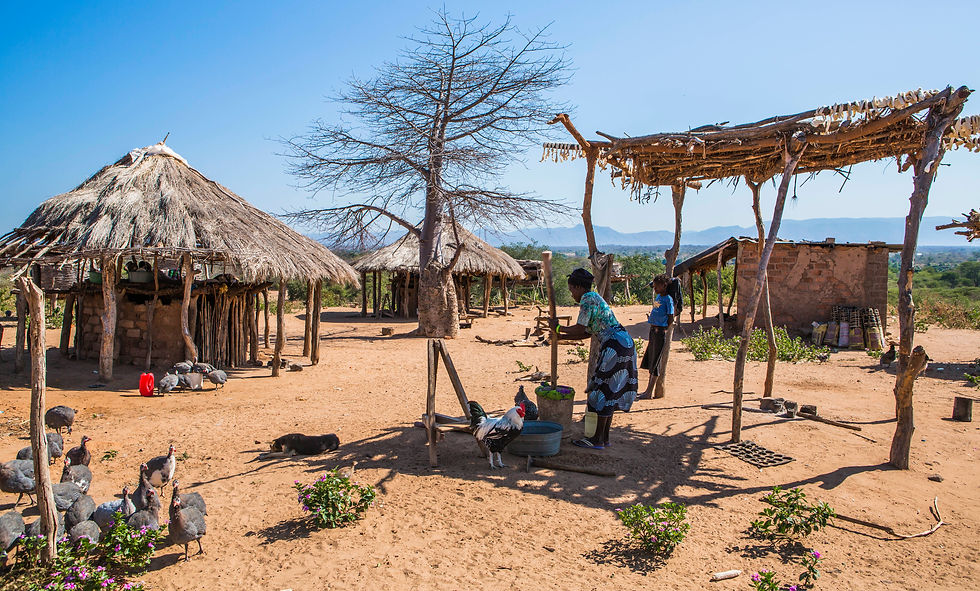
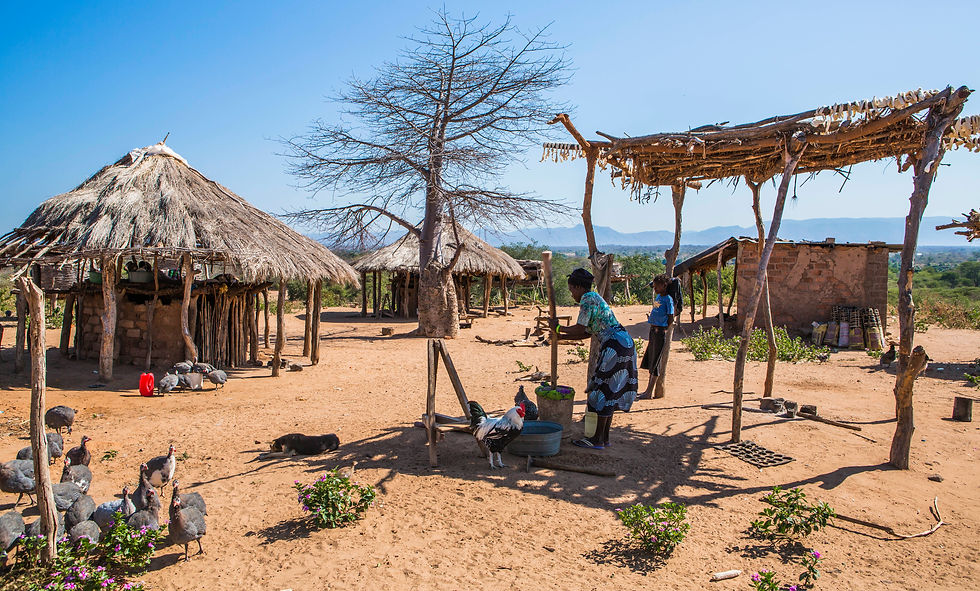
Abstract
In response to worsening food security, developing countries rely on poverty mapping to identify impoverished communities and provide social transfers. Recently, deep learning-extracted satellite features have been used as proxies for household wealth but with a minimal understanding of local poverty. To address these challenges, the proposed study will develop human-interpretable and context-aware wealth metrics to enhance the responsiveness of social safety net programs, specifically the Food Security Pack (FSP), in Zambia. Our AI/ML innovation aims to provide an intuitive, locally relevant, and fine-grained picture of the region’s built environment. We will automatically extract features related to poverty, map their locations, and quantify the contribution of each measured variable. The project is composed of four steps: 1) community engagement to identify visual signs relating to poverty; 2) acquisition and labeling of high-resolution satellite imagery; 3) development of semantic segmentation models to obtain human-interpretable features, such as rooftops and roads; and 4) mapping these features to estimate a granular/community-level of poverty. In doing so, we can increase the poverty-reducing impact of the FSP distribution. This project empowers local residents to conceptualize poverty and assist the government in reaching communities in need.
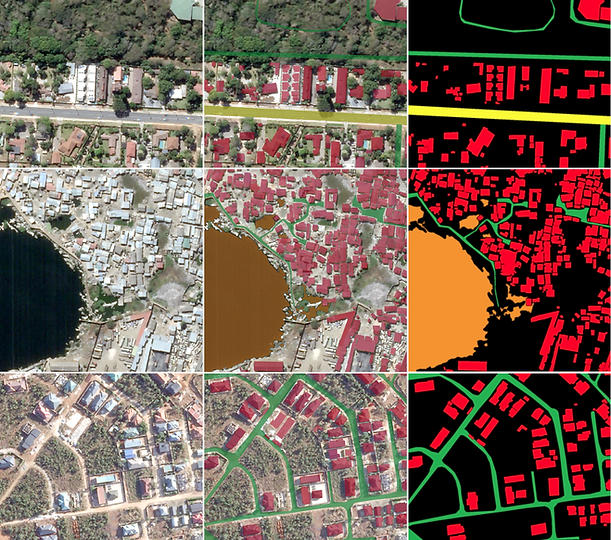
Satellite imaging in Zambia remains largely unexplored, posing distinct challenges for our researchers due to the scarcity of data. To address an absence of pre-annotated images, our team has meticulously examined and annotated more than a thousand Planet Scope images ranging from 50cm to 7m. These efforts aim to equip AI models with the capability to accurately detect poverty-related features across the nation.
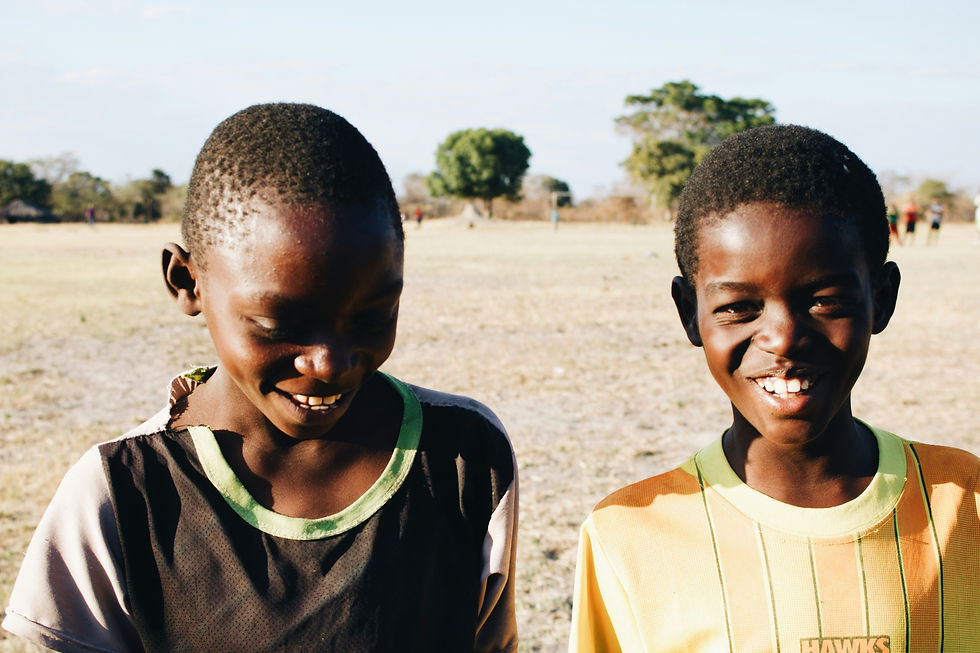
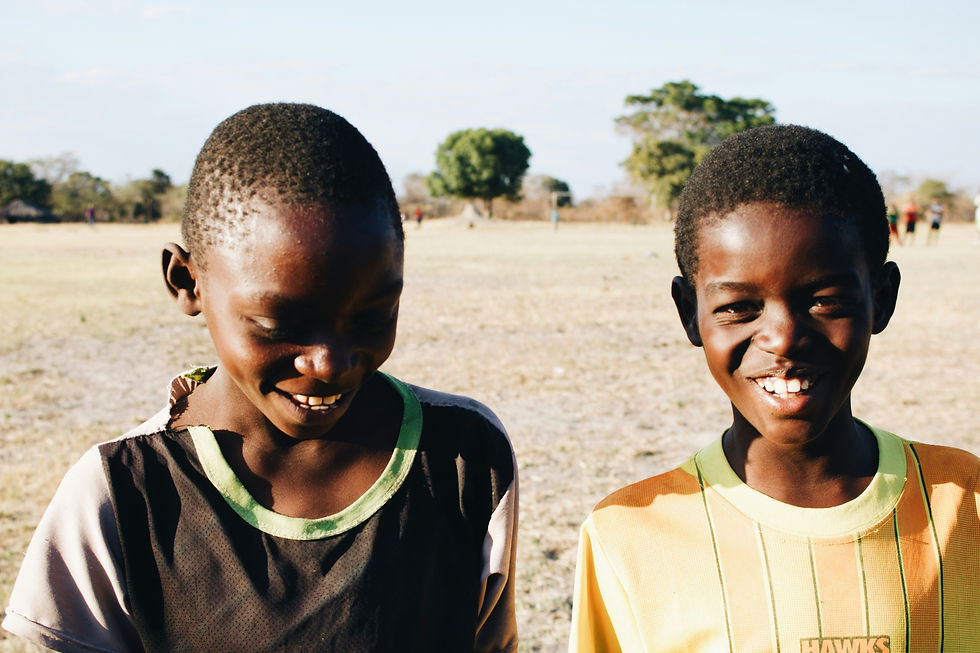
Research
.................................................
Dr. Jung's study aims to enhance the effectiveness of Zambia's Food Security Pack (FSP) program by using AI and Machine Learning innovation to more accurately target vulnerable households.
PARTNERS ....................................................
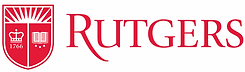
Recipient of the Research Council's Collaborative Multidisciplinary Award
PARTNERS ....................................................
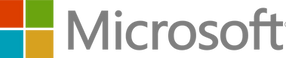
PARTNERS ....................................................

RESEARCH TEAM .................................................................
The team consists of computer/data scientists
and social scientists:
-
Woojin Jung (PI) - Rutgers, School of Social Work
-
Dmitris Metaxas - Rutgers, Computer Science
-
Quentin Stoeffler - University of Bordeaux, Economics
-
Saeed Ghadimi - University of Waterloo, AI Institute
-
Dimitrios Ntarlagiannis - Rutgers, Earth and Env. Sciences
-
Arunesh Sinha - Rutgers, Mgmt Science and Information Systems
-
Min Xu - Rutgers, Statistics
-
Tawfiq Ammari - Rutgers, Communication and Information
-
Andrew Kim - Rutgers, School of Social Work
-
Maryam Hosseini - MIT, Urban Studies and Planning
-
Melissa Sartorius - Rutgers, School of Social Work
Research Duration
.................................................
July 2021 - Ongoing
The study will conclude with the dissemination of findings to policymakers.
4 STAGES ................................................
-
Community engagement to visualize poverty
-
Acquisition and labeling of satellite imagery
-
Development of deep learning models to obtain intuitive features
-
Creation of high-resolution poverty map and index
RESEARCH TEAM .................................................................
-
Sajedeh Goudarzi - Rutgers, Global Urban Studies
-
William Benjamin - Allstate Corp., Data Science
-
Rofaida Benotsmane - Istanbul Technical Univ., Economics
-
Yuxiao Lu - Singapore Management Univ., Computer Science
-
Vatsal Shah - Rutgers, Computer Science, Statistics
-
EJ Knopf - Rutgers, School of Social Work
-
Jamie Cramer - Rutgers, School of Social Work
-
Tamara Billima - Local NGO
-
Bernard Tembo - Local NGO
-
Owen Siyoto - Local NGO
-
Cheelo Mwiinga - Local NGO